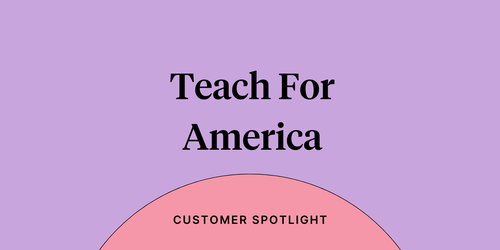
Why your best employees are leaving and how to stop it
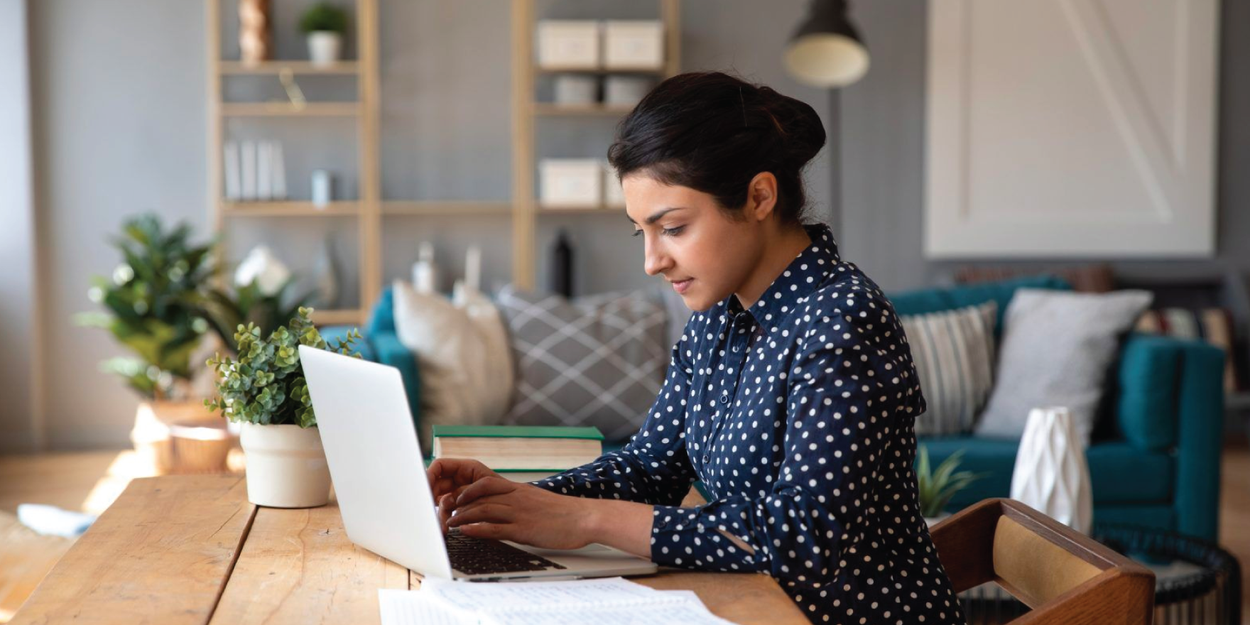
Written by
Director of People Science Research, Culture Amp
Updated (08/27/2021): In the midst of the Great Resignation, we re-ran this analysis with a new cohort of over 100,000 employees who exited between January to August 2021. All of the predictors discussed below remained the same with one important difference. Enablement, specifically not having easy access to the information needed to do one’s job became a new driver of attrition. Crucially this was true across tenures indicating that this is not only a problem for employees who have onboarded remotely but even tenured employees. Organizations working remotely should focus on internal communications and knowledge management to help employees work smoothly while distributed.
Turnover: it’s an important metric for any organization’s health and one that executives across the business often scrutinize. If you feel confused answering questions like, “why are employees leaving,” you’re not alone. It’s hard to know what’s right when the research is flip-flopping, suggesting one minute that employees leave because of managers, and the next, that’s not the case. We at Culture Amp have even weighed in on this debate in the past. If your head is spinning, don’t worry. Ours was, too.
So we undertook our largest study on employee turnover to date, aiming to provide some much-needed context around these challenging questions. We found that:
- There are 3 key reasons employees leave
- When it comes to keeping managers, inclusion is most important
- Employees that you want to keep most are more likely to be vocal about their dissatisfaction
- If you want to predict who is going to leave, you should just ask them
To discover these insights, we analyzed the survey responses of over 300,000 employees who voluntarily exited over the 15 months prior to COVID. We compared both their exit survey responses as well as their engagement survey responses before leaving. Then we incorporated other characteristics we knew about the individuals, like their tenure, manager status, and if the People team considered their exit to be regrettable. We busted some long-established myths – no, employees don’t leave because of managers – and found insights that no one has reported on before. Now, let’s dive in.
There are 3 common reasons employees leave
When comparing those who left to those who stayed, there were three key reasons they left:
- Career growth: Employees who left were less favorable on the question “I believe there are good career opportunities for me at [Company].” Additionally, a lack of Growth (in their career or development opportunities) was selected by 1 in 3 employees as a top reason for leaving at the time of exit.
- Role expectations: Unsurprisingly, they were also less favorable when asked if “I am happy with my role relative to what was described to me.”
- Inclusion: Those who left were less likely to feel like they belonged at the organization.
On average, those who left were 7 points lower on these questions than those who stayed on.
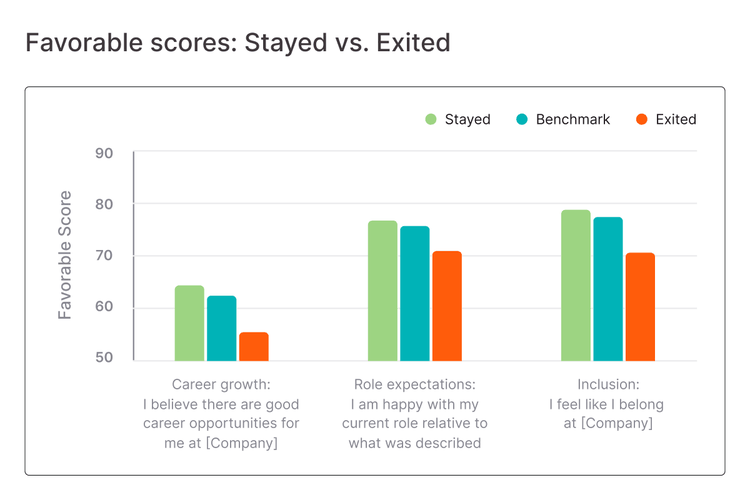
💡 Insight: Your employees are likely not leaving because of their manager, but rather because they feel like they aren’t growing, their role is misaligned, or they don’t feel included at the company.
The same reasons were cited by the employees you want to keep most
While turnover is always difficult, we recognize that not all turnover is created equal. So we looked at 3 key attributes (tenure, manager status, and if their exit was marked as regrettable) to identify employees who have the largest impact when they leave. For each, the same 3 questions mentioned above came up front and center, with a few notable differences.
Career growth was important no matter the tenure
When your most tenured employees leave, they take years of historical knowledge with them, making their departures particularly difficult. Some might assume the need for growth changes over an employee’s tenure. But by looking at the reason cited by employees at the time of exit, we found growth was the number one reason across all tenure groups. This was true for early leavers (those who left in under 1 year), tenured folks (those who stayed for over 4 years), and the in-betweeners. Importantly, growth meant a range of things, not only formal role progression. Many employees cited learning and development opportunities and the desire for stretch projects.
💡 Insight: To keep your employees, no matter the tenure, determine what growth means for each of them.
To keep managers, make sure they feel included
When a manager leaves, it can completely change the dynamic of their team. And it can take longer to hire their replacement than it would for an individual contributor. We found that for managers, the Inclusion aspect was even more important. Managers who left were:
- 9 points lower on “When I share my opinion, it is valued” than managers who stayed
- 11 points lower on “Perspectives like mine are included in decision-making at [Company]”
Perhaps this is because of the classic middle management paradox: managers need to lead and follow. If they don’t feel they can be a good representative or taken seriously while advocating for their team, they choose to go somewhere else.
💡 Insight: To stop manager turnover, be sure to ask managers (and everyone!) if they feel like they have a voice and are included in decision-making.
Employees whose exit was marked as “regrettable” were even more honest
For about 10,000 employees, the People team had flagged their exit as “regrettable.” This definition varies by company but generally means the individual was high-performing and the company wishes they could have retained them. Surprisingly, we found that these employees were much more honest in their dissatisfaction than those who exited and were not marked regrettable – a full 10 points lower in overall favorability.
In the table below, you can see this pattern for the 3 main drivers of attrition. The favorable scores of employees marked as regrettable exits were low enough to be placed within the bottom 10th percentile of our global benchmark data.
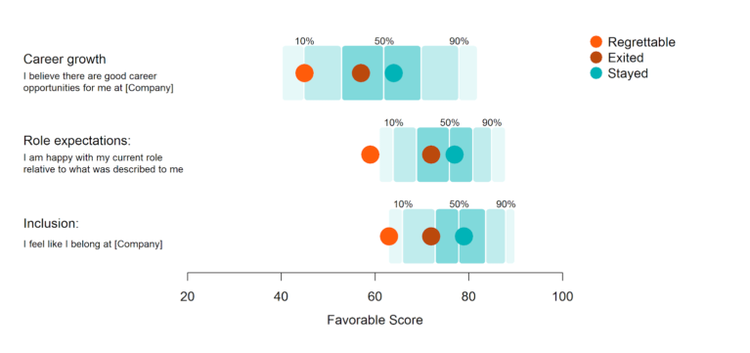
💡 Insight: If you have low scores on your Engagement survey, it can be easy to question who the unfavorable folks are. This research indicates they might be the very employees you most want to keep!
The best way to predict who is going to leave? Just ask them
Of all the questions we looked at, the most predictive by far was our future commitment item – “I see myself still working at [Company] in two years’ time.”
- Those who strongly disagreed were 2.7x more likely to leave than those who selected other response options. This effect was twice as strong for regrettable exits since they are more honest about their intentions!
- Those who disagreed were ~2x times more likely to leave than those who were neutral or agreed.
- And even those who were neutral were 50% more likely to leave than those who agreed.
This result is consistent with our 2017 findings.
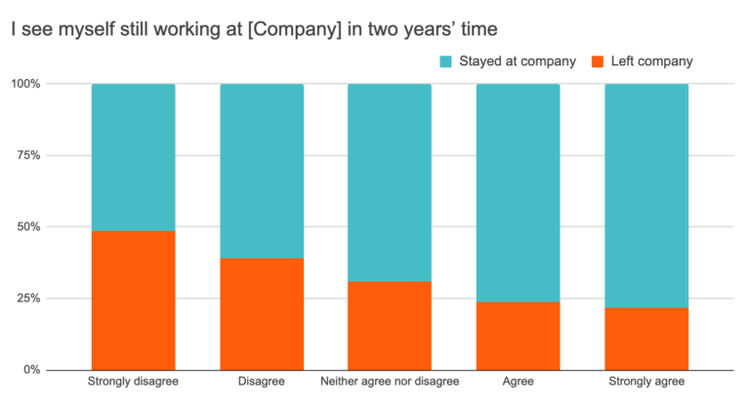
💡 Insight: Asking your employees if they intend to stay at the company is the best way to predict attrition. Use the proportions above for a rough forecast.
Employees will also tell you why they plan to leave
In our exit survey, employees are asked for the top reason they chose to leave. We found the reason selected at the time of exit was highlighted in their Engagement survey responses months before.
- Regrettable employees who selected “Manager” as their key reason for leaving scored below the bottom 5th percentile on the Management factor in their Engagement response. This was a full 29 points below regrettable employees who did not name their manager as a reason for leaving.
- Similarly, employees who selected “Leadership” as their key reason for leaving scored below the bottom 5th percentile on the Leadership factor.
- A similar signal was found within the compensation question “I believe my total compensation is fair, relative to similar roles at other companies”
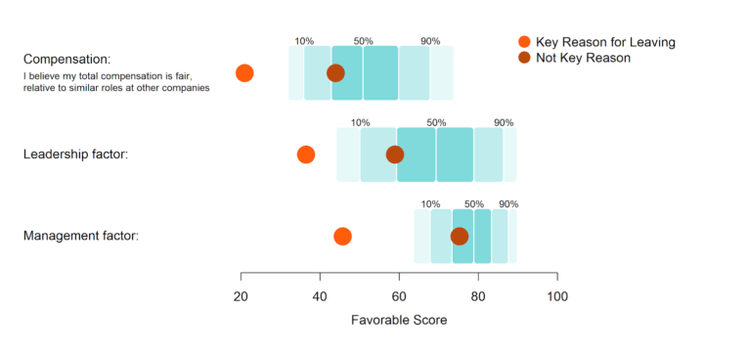
💡 Insight: If employees are vocal about their dissatisfaction on particular topics and the problem isn’t addressed, they will leave because of it.
Take your first step
Based on our research, you can be confident that:
- Your employees will tell you if they’re planning to leave
- They’ll let you know why they’re planning to leave
- The employees you most want to keep (regrettable exits) are even more honest with their feedback
However, our research was easy since employees were flagged as regrettable by the People team. Unfortunately, this only happens once an employee leaves. To get ahead of the curve:
- Include performance rating as a filter in your Engagement survey results so that you can see how your high-performing employees’ experiences may differ.
- Be sure to ask the most predictive questions. Both by asking directly, “I see myself still working at [Company] in two years’ time,” and indirectly by covering Career growth, Role expectations, and Inclusion.
If you do, you just might be able to stop your high-performing employees before they make that pivotal decision.
At Culture Amp, much of these insights around engagement-related turnover are already embedded within our product. We do the heavy lifting to make it easy for you to identify turnover risks and improve their experience before it’s too late.